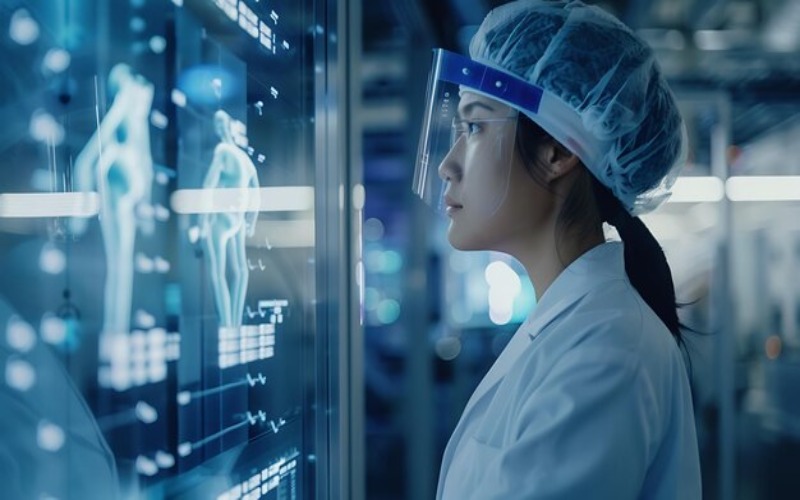
[ad_1]
“It isn’t the strongest of the species that survives, nor the most clever, however the one most responsive to vary.” – Charles Darwin
The healthcare trade has been on the forefront of the AI revolution. The advantages of AI are simple to see in healthcare – serving to sufferers overcome well being literacy, sooner R&D and faster completion of medical trials, simpler prognosis of uncommon illnesses, fewer errors in affected person knowledge alternate throughout organizations and computing programs, and the automation of time-consuming enterprise processes – all feeding within the utopian objectives of discount in prices and dangers whereas growing adherence to regulatory compliance.
AI Rules – Present State & Gaps
An absence of clear regulation that governs using AI in healthcare is limiting its development. Generally, healthcare has been one of the regulated industries with frameworks such because the FDA GxP pointers, as encapsulated in 21 CFR Half 11. These rules encourage good practices, enhance transparency, and make varied stakeholders extra accountable for managing affected person well being. Sadly, regulatory regimes have didn’t preserve tempo with technological development, significantly AI.
Exacerbating the issue is the mushrooming of startups centered on making use of AI to completely different facets of the healthcare worth chain. With the assist of angel traders and enterprise capital funds, greater than 4,000 AI startups launched within the final two years, and analysts anticipate 10,000 extra to start out within the coming years. With a lot innovation and funding occurring, it’s much more crucial to deal with the legitimate considerations concerning bias in coaching knowledge, hallucination and likelihood of incorrect outcomes, knowledge privateness and knowledge safety, lack of requirements round ethics throughout the areas, interoperability of knowledge between healthcare programs, amongst others.
Within the absence of federal requirements, many states have proposed separate AI payments and rules, which, if handed, would create something however a holistic method important to drive trade adoption. Lately, California, one of many largest tech hubs in America, is attempting to fix these points with SB 1047 and AB 2013, AI regulation payments that at present sit on the desk of California Governor Gavin Newsom. SB 1047 shouldn’t be solely an bold invoice designed to implement security requirements for the event of enormous AI fashions, nevertheless it might additionally act as a bellwether for future AI regulation and have an effect on the way forward for healthcare in the USA.
Within the international enviornment, the European Union has taken the lead in defining AI rules as a part of the AI Act in March 2024. Beneath the Act, any AI system that may be a Class IIa (or increased) medical gadget or makes use of an AI system as a security element is designated as “excessive threat.” Such programs might want to adjust to a raft of further necessities, lots of which overlap with the present rigorous necessities of conformity evaluation beneath the EU MDR and IVDR. Nonetheless, the AI Act doesn’t cowl using AI in lots of essential areas, corresponding to drug discovery, medical trial recruitment, pharmacovigilance, and member enrollment.
There’s additionally a scarcity of clear pointers on the applicability of the audit and CSV necessities (corresponding to IQ/OQ/PQ processes) which can be a part of 21 CFR Half 11 for the AI programs in healthcare.
Bias & Incorrect Information Units For Coaching
One other key problem is the inherent bias and incorrect info within the obtainable dataset.
One of the vital seen instances highlighting this problem is analysis by Derek Driggs, an ML researcher on the College of Cambridge. Driggs constructed an ML mannequin for illness prognosis with higher accuracy than physicians. Nonetheless, additional investigation revealed that his mannequin was flawed as a result of it was skilled on a knowledge set that included scans of sufferers mendacity down and standing up. The sufferers mendacity down had been more likely to be severely ailing, so the algorithm discovered to determine illness threat based mostly on the particular person’s place within the scan.
This case sounds eerily like one other well-known experiment the place an AI mannequin discovered to find out whether or not the photographs depicted wolves or huskies by trying to find snow because the authentic coaching datasets for the algorithm included a disproportionate variety of photographs of wolves in winter as an alternative of zeroing in on the animals and their variations.
These examples should not one-off instances. Bias and incorrect knowledge units are intrinsic and fixed dangers for each AI mannequin. A sturdy AI framework would assist construct transparency and predictability the place sufferers and regulators can belief the AI fashions.
AI-Pushed Diagnostics, Automation, Drug-Discovery:
A number of different use instances have emerged the place AI is utilized in affected person care, illness prognosis, workflow automation, drug discovery, and plenty of extra. These use instances can primarily be categorized as areas the place AI is primarily utilized to extend the velocity and accuracy of present work patterns. Let’s have a look at a couple of of those use instances:
- Diagnosing Sufferers: AI algorithms analyze medical imaging knowledge, corresponding to X-rays, MRIs, and CT scans, to help healthcare professionals in correct and swift diagnoses.
- Transcribing Medical Paperwork: Automated Speech Recognition (ASR) expertise employs superior algorithms and machine studying fashions to transform spoken language into written textual content, offering a extra environment friendly and correct methodology for documenting medical info.
- Drug Discovery and Improvement: AI accelerates the drug discovery course of by analyzing huge datasets to determine potential drug candidates and predict their efficacy.
- Administrative Effectivity: AI streamlines administrative duties, corresponding to billing and scheduling, lowering paperwork and bettering general operational effectivity inside healthcare organizations.
Whereas the quantity and utilization of use instances are growing, a elementary paradox is on the core right here.
The present method to healthcare regulation is based on predictability. Regulators just like the FDA assessment the approval request for medicine and gadgets (NDA/BLA/PMA) based mostly on the security and efficacy knowledge generated within the managed trials.
Nonetheless, the world of AI is based on fixed coaching with new datasets. Limiting the AI mannequin to a managed dataset or constrained setting defeats the aim of the machine studying functionality that makes AI efficient. AI fashions are anticipated to vary their response to the identical query as they be taught from the minutest of the variations within the surrounding setting.
These two approaches are at odds with one another. With no clear regulatory framework, the sponsors and customers of the AI use case assume legal responsibility and model threat. That is actually a brand new frontier, and we want breakthroughs in well being coverage as dramatic as AI.
Conclusion
The World Well being Group’s current publication, which lists key regulatory concerns on synthetic intelligence (AI) for well being, generally is a good begin to untangle these complicated points. The 18 regulatory concerns mentioned within the publication fall beneath six broad classes: documentation and transparency, threat administration, supposed use and validation, knowledge high quality, privateness and knowledge safety, and engagement and collaboration.
It’s as much as the trade, regulators, academia, legislators, and affected person organizations to return collectively to make sure that AI delivers on the promise of a greater (and more healthy) world.
[ad_2]
Supply hyperlink